DeepSeek-V3 vs Deepseek R1: What’s the Differences?
DeepSeek, a prominent Chinese AI startup, has introduced two notable models—DeepSeek-V3 and DeepSeek-R1—that have garnered significant attention in the artificial intelligence community. While both models stem from the same organization, they are tailored for distinct applications and exhibit unique characteristics. This article provides an in-depth comparison of DeepSeek-V3 and R1, examining their architectures, performance, applications, and the implications of their emergence in the AI landscape.
What Are DeepSeek-V3 ?
DeepSeek-V3 is a general-purpose LLM aimed at delivering balanced performance across diverse tasks. The initial version, released in December 2024, featured 671 billion parameters. In March 2025, an updated version, DeepSeek-V3-0324, was introduced with 685 billion parameters, employing a Mixture of Experts (MoE) architecture that activates approximately 37 billion parameters per token. This enhancement has led to significant improvements in code generation, reasoning, mathematics, and Chinese language processing capabilities.
Related topics DeepSeek V3-0324 Release: What Are Its Latest Enhancements?
What Are DeepSeek-R1?
DeepSeek-R1, released in January 2025, is tailored for tasks requiring advanced reasoning and complex problem-solving, particularly excelling in mathematics and coding. It builds upon the DeepSeek-V3 framework, incorporating multi-head latent attention and MoE to reduce key-value cache requirements and enhance inference efficiency.
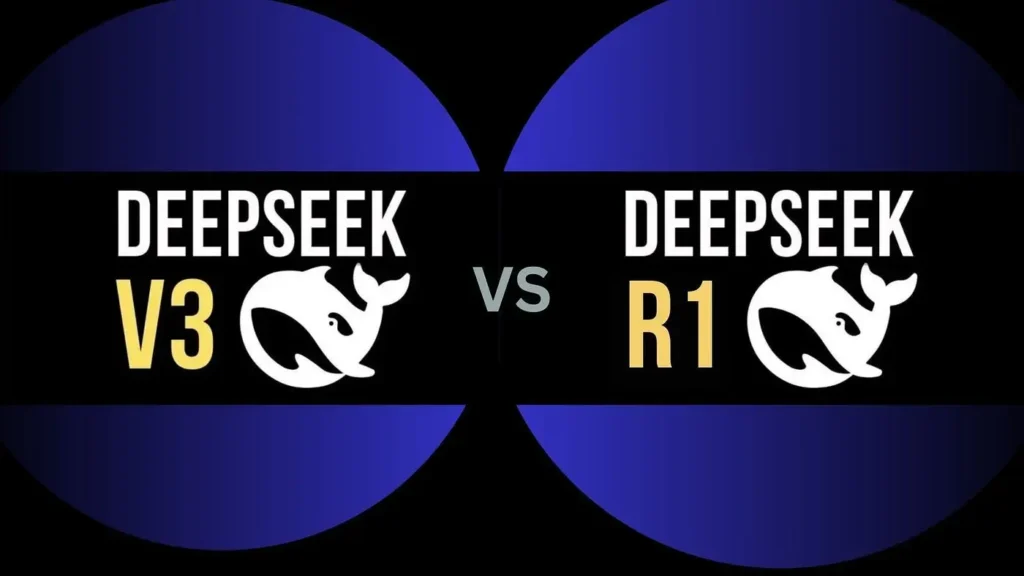
What Are the Core Differences Between DeepSeek-V3 and R1?
DeepSeek R1 vs V3: Core Differences
Here’s a table comparing DeepSeek R1 vs. DeepSeek V3: Core Differences:
Feature | DeepSeek R1 | DeepSeek V3 |
---|---|---|
Processing Speed | Optimized for fast response times and efficiency | Slightly slower but more accurate in complex tasks |
Language Comprehension | Strong, with focus on clear, concise outputs | Enhanced, with deeper understanding of context and nuance |
Architecture | Reinforcement Learning (RL) optimized | Mixture-of-Experts (MoE) |
Reasoning Ability | Good, focuses on structured tasks | Advanced reasoning and problem-solving capabilities |
Training Dataset | Reinforcement learning for reasoning | Coding, mathematics, multilingualism |
Real-World Applications | Well-suited for quick content generation, coding tasks | Better suited for research, complex analysis, and nuanced interactions |
Customization | Limited customization options | More flexible, allowing deeper customization for specific tasks |
Latency | Low latency, high-speed performance | Slightly higher latency due to more processing power required |
Best Use Case | Ideal for tasks requiring speed and accuracy | Best for tasks needing in-depth understanding and reasoning |
Parameter Range | 1.5B to 70B | 671B |
Open Source | Yes | Yes |
Architectural Distinctions
DeepSeek-V3 is designed as a general-purpose AI model, emphasizing versatility and broad applicability across various tasks. Its architecture focuses on delivering balanced performance, making it suitable for applications requiring a wide range of functionalities. In contrast, DeepSeek-R1 is optimized for tasks demanding advanced reasoning and complex problem-solving capabilities, particularly excelling in areas such as mathematics and coding. This specialization is achieved through targeted training methodologies that enhance its proficiency in handling intricate computations and logical deductions.
Performance Metrics
In benchmark evaluations, DeepSeek-R1 has demonstrated superior performance in tasks involving deep reasoning and complex problem-solving compared to DeepSeek-V3. For instance, in mathematical problem-solving scenarios, R1’s advanced reasoning capabilities enable it to outperform V3, which is more attuned to general tasks. However, V3 maintains an edge in tasks requiring natural language processing and general comprehension, where its balanced approach allows for more coherent and contextually relevant responses.
How Do Training Methodologies Differ Between the Two Models?
Resource Allocation and Efficiency
DeepSeek-R1’s development involved the use of approximately 2,000 Nvidia H800 chips, with a total expenditure of around $5.6 million. This efficient resource utilization contrasts sharply with the substantial investments typically associated with models like OpenAI’s GPT-4, which can exceed $100 million in training costs. The strategic allocation of resources in R1’s training underscores DeepSeek’s commitment to cost-effective AI development without compromising performance.
Training Techniques
Both models employ innovative training techniques to enhance their capabilities. DeepSeek-R1 utilizes methods such as knowledge distillation and a system of specialists to refine its reasoning abilities, enabling it to tackle complex tasks with greater accuracy. DeepSeek-V3, while also incorporating advanced training methodologies, focuses on achieving a balance between versatility and performance, ensuring its applicability across a broad spectrum of tasks.
Related topics How Did DeepSeek Achieve Such Cost-Effective AI Training?
What Are the Practical Applications of Each Model?
DeepSeek-V3: Versatility in Action
DeepSeek-V3’s general-purpose design makes it suitable for a wide array of applications, including:
- Customer Service: Providing coherent and contextually relevant responses to customer inquiries across various industries.
- Content Generation: Assisting in drafting articles, blogs, and other written materials by generating human-like text.
- Language Translation: Facilitating accurate and nuanced translations between multiple languages.
Its balanced performance across diverse tasks positions V3 as a reliable tool for applications requiring a broad understanding and adaptability.
DeepSeek-R1: Specialization in Complex Tasks
DeepSeek-R1’s specialized architecture makes it particularly effective in domains such as:
- Education: Providing detailed explanations and solutions for complex mathematical and scientific problems, aiding both students and educators.
- Engineering: Assisting engineers in performing intricate calculations and design optimizations.
- Research: Supporting researchers in data analysis and theoretical explorations that require deep reasoning.
Its proficiency in handling tasks that demand advanced reasoning underscores its value in specialized fields requiring high levels of cognitive processing.
How Has the Emergence of DeepSeek-V3 and R1 Impacted the AI Industry?
Disruption of Established Players
The introduction of DeepSeek’s models has significantly disrupted the AI landscape, challenging the dominance of established entities like OpenAI and Google. DeepSeek-R1, in particular, has demonstrated that high-performance AI models can be developed with considerably lower financial and computational resources, prompting a reevaluation of investment strategies within the industry.
Market Dynamics and Investment Shifts
The rapid ascent of DeepSeek’s models has influenced market dynamics, leading to notable financial implications for major tech companies. For instance, the popularity of DeepSeek’s AI applications contributed to a significant decrease in Nvidia’s market capitalization, highlighting the profound impact of cost-effective AI solutions on the broader technology market.
How much DeepSeek-V3 and DeepSeek-R1 cost?
DeepSeek offers API access to its models, DeepSeek-Chat (DeepSeek-V3) and DeepSeek-Reasoner (DeepSeek-R1), with pricing based on token usage. The rates vary depending on the time of day, with standard and discounted periods. Below is a detailed breakdown of the pricing structure:
Model | Context Length | Max CoT Tokens | Max Output Tokens | Time Period (UTC) | Input Price (Cache Hit) | Input Price (Cache Miss) | Output Price |
---|---|---|---|---|---|---|---|
DeepSeek-Chat | 64K | N/A | 8K | 00:30-16:30 | $0.07 per 1M tokens | $0.27 per 1M tokens | $1.10 per 1M tokens |
16:30-00:30 | $0.035 per 1M tokens | $0.135 per 1M tokens | $0.55 per 1M tokens | ||||
DeepSeek-Reasoner | 64K | 32K | 8K | 00:30-16:30 | $0.14 per 1M tokens | $0.55 per 1M tokens | $2.19 per 1M tokens |
16:30-00:30 | $0.035 per 1M tokens | $0.135 per 1M tokens | $0.55 per 1M tokens |
Notes:
CoT (Chain of Thought): For DeepSeek-Reasoner, the CoT refers to the reasoning content provided before delivering the final answer. The output token count includes both the CoT and the final answer, and they are priced equally.
Cache Hit vs. Cache Miss:
- Cache Hit: Occurs when the input tokens have been previously processed and cached, resulting in a lower input price.
- Cache Miss: Occurs when the input tokens are new or not found in the cache, leading to a higher input price.
Time Periods:
- Standard Price Period: 00:30 to 16:30 UTC.
- Discount Price Period: 16:30 to 00:30 UTC. During this time, discounted rates are applied, offering significant cost savings.
DeepSeek reserves the right to adjust these prices, so users are encouraged to monitor the official documentation for the most current information.
By understanding this pricing structure, developers and businesses can effectively plan and optimize their usage of DeepSeek’s AI models to suit their specific needs and budgets.
For Developers: API Access
CometAPI offer a price far lower than the official price to help you integrate DeepSeek V3 API (model name: deepseek-v3;) and DeepSeek R1 API (model name: deepseek-r1;), and you will get $1 in your account after registering and logging in! Welcome to register and experience CometAPI.
CometAPI acts as a centralized hub for APIs of several leading AI models, eliminating the need to engage with multiple API providers separately.
Please refer to DeepSeek V3 API and DeepSeek R1 API for integration details.
Conclusion
DeepSeek-V3 and R1 exemplify the innovative strides being made in the field of artificial intelligence, each catering to distinct needs within the technological ecosystem. V3’s versatility makes it a valuable asset for general applications, while R1’s specialized capabilities position it as a formidable tool for complex problem-solving tasks. As these models continue to evolve, they not only enhance the scope of AI applications but also prompt a reevaluation of development strategies and resource allocations within the industry. Navigating the challenges associated with their deployment will be crucial in determining their long-term impact and success in the global AI landscape.