How Much Did It Cost to Train GPT-4o? (exposed!)
OpenAI‘s GPT-4o represents a significant advancement in artificial intelligence, offering enhanced capabilities across text, image, and audio processing. Understanding the costs associated with GPT-4o involves examining both the expenses incurred during its development and training, as well as the pricing models implemented for end-users.
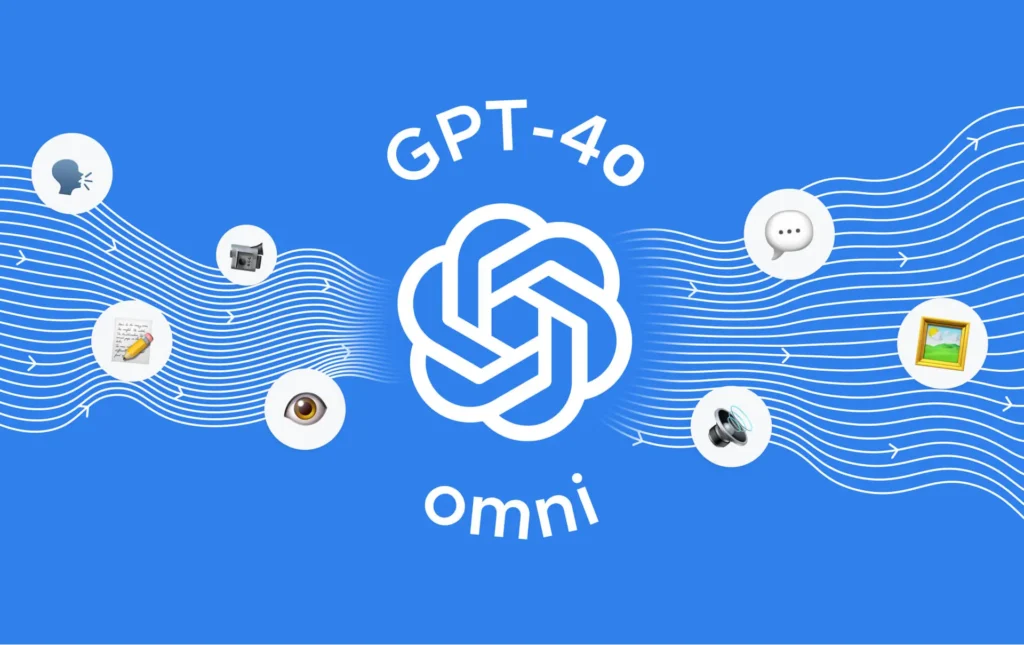
What is GPT-4o ?
GPT-4o, where “o” stands for “omni,” is OpenAI’s advanced multimodal AI model introduced in May 2024. This model is designed to process and generate various forms of data, including text, audio, images, and video, facilitating more natural and dynamic human-computer interactions.
What Are the Training Costs Associated with GPT-4o?
Training state-of-the-art AI models demands significant computational resources, extensive datasets, and considerable time, all contributing to high financial outlays.
Estimated Expenses for Training GPT-4o
While OpenAI has not publicly disclosed the exact cost of training GPT-4o, insights can be gleaned from comparable models. For instance, OpenAI’s GPT-4 model, launched in late 2023, reportedly cost over $100 million to train. This figure underscores the substantial investment required for developing such advanced AI systems.
Factors Influencing Training Expenses
Several key components contribute to the overall cost of training advanced AI models:
- Computational Resources: High-performance GPUs or TPUs are essential for processing vast datasets, representing a significant portion of the expenditure.
- Data Acquisition and Storage: Curating and storing extensive datasets necessary for training adds to the financial outlay.
- Research and Development: The expertise required to design, implement, and fine-tune complex models incurs considerable costs.
- Operational Expenses: Costs related to electricity, cooling systems, and maintenance of data centers also contribute to the total investment.
It’s important to note that cost estimates can vary widely based on the model’s architecture, the scale of training data, and the efficiency of the training process.
Variability in Cost Estimates
It’s important to note that cost estimates can vary widely based on the model’s architecture, the scale of training data, and the efficiency of the training process. Reports suggest that training models comparable to GPT-4 have seen costs decrease to approximately $100 million, highlighting advancements in training efficiency.
How Is GPT-4o Priced for End-Users?
OpenAI has adopted a tiered pricing model for GPT-4o, offering various subscription plans to cater to different user needs.
Subscription Tiers and Associated Costs
- ChatGPT Plus: Priced at $20 per month, this plan provides users with access to GPT-4o’s advanced features, including enhanced image generation capabilities.
- ChatGPT Pro: At $200 per month, the Pro tier offers unlimited access to premium models such as OpenAI o1, GPT-4o, and Advanced Voice mode. This subscription is designed for users requiring extensive computational resources and advanced functionalities.
API Access and Usage-Based Pricing
For developers and enterprises seeking to integrate GPT-4o into their applications, OpenAI provides API access with usage-based pricing. The cost structure for API usage is as follows:
- GPT-4o: $2.50 per million input tokens and $10 per million output tokens.
- GPT-4o Mini: A more affordable variant, GPT-4o Mini, is available at $0.15 per million input tokens and $0.60 per million output tokens. This model is particularly suited for startups and developers requiring cost-effective solutions.
Free Access Limitations
OpenAI also offers limited free access to GPT-4o’s features. For instance, users can generate up to three images per day without a subscription. However, due to high demand and associated computational costs, free access is subject to restrictions.
Access GPT-4o API in CometAPI:
CometAPI provides access to over 500 AI models, including open-source and specialized multimodal models for chat, images, code, and more. Its primary strength lies in simplifying the traditionally complex process of AI integration. With it, access to leading AI tools like Claude, OpenAI, Deepseek, and Gemini is available through a single, unified subscription.
You can use the API in CometAPI to create music and artwork, generate videos, and build your own workflows. CometAPI offer a price far lower than the official price to help you integrate GPT-4o API (model name: gpt-4o-all), and you will get $1 in your account after registering and logging in! Welcome to register and experience CometAPI.CometAPI pays as you go,GPT-4o API in CometAPI Pricing is structured as follows:
- Input Tokens: $2 / M tokens
- Output Tokens: $8 / M tokens
Please refer to GPT-4o API and GPT-4.5 API for integration details.
How Do Training Costs Impact the AI Industry?
The substantial investments required for training advanced AI models have several implications for the industry:
- Barrier to Entry: High costs may limit the ability of smaller organizations and startups to develop cutting-edge models, potentially leading to a concentration of AI advancements within well-funded tech giants.
- Innovation in Efficiency: The financial demands drive research into more efficient training methods, aiming to reduce costs without compromising performance.
- Open-Source Contributions: Collaborative efforts within the open-source community have been instrumental in developing tools and techniques that lower training expenses, democratizing access to AI technologies.
Case Study: DeepSeek’s Cost-Efficient Model Training
An illustrative example of cost reduction in AI training is provided by the Chinese AI startup DeepSeek. The company reportedly trained a model comparable to leading AI systems for approximately $5.6 million, significantly less than the typical expenditures exceeding $100 million by U.S. counterparts. This development has prompted discussions about the potential for more cost-effective AI model training and its impact on the competitive landscape.
What Strategies Are Employed to Mitigate Training Costs?
Organizations adopt various approaches to manage and reduce the expenses associated with training large AI models:
- Utilizing Pre-trained Models: Leveraging existing models and fine-tuning them for specific applications can be more cost-effective than training from scratch.
- Optimizing Algorithms: Developing more efficient algorithms that require less computational power can lead to significant cost savings.
- Cloud Computing Services: Renting computational resources from cloud providers offers scalability and reduces the need for substantial upfront investments in hardware.
- Collaborative Research: Engaging in partnerships and contributing to open-source projects can distribute the financial burden and foster innovation.
What Are the Environmental and Operational Costs Associated with GPT-4o?
Beyond financial considerations, operating models like GPT-4o incurs environmental and operational costs:
Computational Demand and Energy Consumption
The deployment of GPT-4o has led to substantial strain on computational resources. OpenAI’s CEO, Sam Altman, noted that the overwhelming demand for image generation caused GPUs to “melt,” necessitating temporary limitations on image generation requests to maintain system stability.
Sustainability Challenges
The extensive computational power required by GPT-4o raises concerns about its environmental footprint. AI data centers consume significant energy for both processing and cooling, prompting discussions about the sustainability of such technologies. Efforts are underway to explore more efficient cooling methods and the use of renewable energy sources to mitigate these impacts.
Addressing these challenges is crucial for the responsible and sustainable development of AI technologies.
Conclusion
While the exact cost of training OpenAI’s GPT-4o remains undisclosed, insights from similar models indicate that such endeavors require multi-million-dollar investments. These substantial costs underscore the need for ongoing research into more efficient training methodologies and highlight the importance of collaborative efforts to make advanced AI technologies more accessible across the industry.